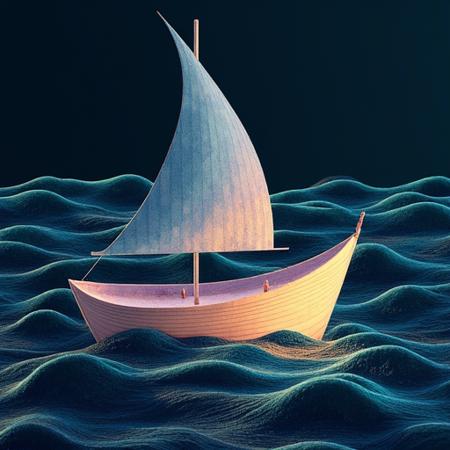
The pro-positioning system (attempted in the demo prompts) is ***NOT FUNCTIONAL***, so you can't say "Enhance 5,5 by .5"... yet, back to the drawing board- I am not super-smart so this may be an ignorant way to approach this lol.
-
My code created the dataset images with metadata (it may need more) and then I captioned the images with the AI generated info with the precise positioning data. I will try a natural language version next, probably.
GPT$:
I can generate accurate descriptions based on metadata to enhance text-to-image models like LoRA. To proceed:
1. Extract Metadata: I'll analyze the details from your uploaded images or datasets to generate precise positioning-related descriptions.
2. Generate Descriptions: I'll craft metadata-based positional and compositional descriptions to refine LoRA's text2img capabilities.
Let me start by examining the contents of the uploaded file.
The folder contains paired JSON and PNG files, likely representing metadata and corresponding images. I will:
1. Inspect Metadata (JSON files): Extract relevant positioning data to craft descriptive prompts.
2. Link Metadata to Images: Ensure descriptions align with the visual content.
I'll start by examining one JSON file to understand its structure.
The JSON file contains metadata attributes, including:
- Shape: "cone"
- Size: Relative size of the object.
- Color: RGB color values.
- Position: X, Y, Z coordinates.
- Rotation: Rotational angles in degrees.
- Wireframe: Boolean for display style.
- Transparency: Transparency level.
- Environmental Context: "perlin"
(likely a texture or background type).
- Layering: Boolean and layer count.
- Shadows: Boolean for shadow inclusion.
- Sequence ID: Identifier for the image.
I'll use these details to create a detailed and descriptive prompt for LoRA text-to-image training. Let's begin with this sample file.
Here is a descriptive prompt based on the metadata:
Prompt: "A cone object with a size of 0.82, colored in RGB(174, 140, 149), positioned at coordinates (X: 0.41, Y: 1.62, Z: -0.75). The object is rotated to angles (Yaw: 197.78°, Pitch: 137.22°, Roll: 263.72°), and displayed in a wireframe style with a transparency level of 0.5. The environment is 'perlin' with layering enabled and a layer count of 2. Shadows are enabled."
This style of description can be generated for all the metadata files to enhance text-to-image training precision.
---
### It may have learned something about 3d perspectives in images but I need to test. Also this was just trained on 50 images of cones but I have other shapes too and I will try to build a larger more functional dataset possibly on a different track.
Example from my python code dataset.
描述:
You can't say "Place {object} at .05, .05, .05" yet, sorry.
训练词语:
名称: 1068823_training_data.zip
大小 (KB): 3334
类型: Training Data
Pickle 扫描结果: Success
Pickle 扫描信息: No Pickle imports
病毒扫描结果: Success
名称: Image_Positioner_AIpro.safetensors
大小 (KB): 18833
类型: Model
Pickle 扫描结果: Success
Pickle 扫描信息: No Pickle imports
病毒扫描结果: Success