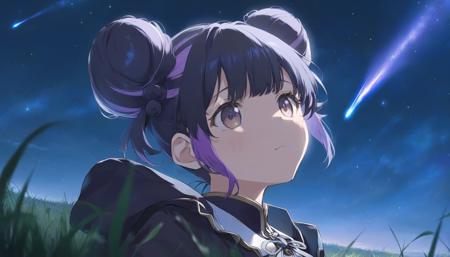
Full Report:
https://nieta-art.feishu.cn/wiki/PpwqwVDzjiNE5kkUhRtcEsn6nmh
First release address:
Civitai - https://civitai.com/models/410737
Liblib - https://www.liblib.art/modelinfo/55b06e35dd724862b3524ff00b069fe8
I. Overview
-
We have updated Neta Art XL V2.0. Comparing to version 1.0, we have optimized the character's posture dynamics, further strengthened hand stability, and are very good at telling stories with atmospheric epic scenes.
-
Main motivation:
-
In V1.0, the default poses generated by our characters are relatively fixed, while the new version enhances the sense of dynamics, diverse character camera expressions, and more precise prompt control.
-
On the basis of increasing more stylistic diversity, more stable anatomy has been maintained, especially the probability of having five fingers (instead of four or six) on the hands is now higher!
-
Further training methods for issues such as Rectified Flow and Noise Offset have been better deployed. The current picture has a strong sense of epic, and can now show very bright and dark scenery (as shown in the figure below).
-
{
"prompt": "1girl, full moon, moonlight shadow, cinematic lighting, battle field, fighting armies, castle, warriors around, waving, looking at viewer, Heterochromatic pupil, sitting on a cliff, very dark, epic scene, inferno, cowboy shot, depressed, angel wings, solo, white long hair, wings bangs, beautiful color, amazing quality,",
"negative_prompt": "nsfw, lowres, bad anatomy, bad hands, text, error, missing fingers, extra digit, fewer digits, cropped, worst quality, low quality, normal quality, jpeg artifacts, signature, watermark, username, blurry, artist name, rating: sensitive, low contrast, signature, flexible deformity, abstract, low contrast, ",
"resolution": "1344 x 768",
"guidance_scale": 8,
"num_inference_steps": 28,
"sampler": "Euler a",
"use_lora": null,
"use_upscaler": {
"upscale_method": "bislerp",
"upscaler_strength": 0.65,
"upscale_by": 1.5,
"upscale_cfg": 11,
"new_resolution": "2016 x 1152"
}
}
Prompt guide
In Neta V2, we re-trained based on ArtiWaifu Diffusion V1.0 (AWA1). Therefore, we used the prompt order summarized in ArtiWaifu:
Tag order: art style ( by xxx ) - > character ( 1 frieren (sousou no frieren) ) - > race (elf) - > composition (cowboy shot) - > painting style ( impasto ) - > theme (fantasy theme) - > main environment (in the forest, at day) - > background (gradient background) - > action (sitting on ground) - > expression (expressionless) - > main characteristics (white hair) - > other characteristics (Twintails, green eyes, parted lips) - > clothing (wearing a white dress) - > clothing accessories (frills) - > other items (holding a magic wand) - > secondary environment (grass, sunshine) - > aesthetics ( beautiful color , detailed ) - > quality ( best quality) - > secondary description (birds, clouds, butterflies)
V. Training strategies
Model Training is divided into three stages.
-
Rough practice: Teach models basic knowledge and concepts of the second dimension. Learning Rate: Constants 1e-5 (unet), 7.5e-6 (text encoder) Equivalent batch size: 96 Optimizer: AdaFactor (False relative_step, scale_parameter and warmup_init) Noise-free offset Time step sampling: LogitNormal (ln3,1) Minimum signal to noise ratio: 5 Note: Since the basic model has most of the prior knowledge, this stage is skipped in actual training.
-
Concept Reinforcement: This stage teaches and reinforces all the concepts that the model needs to learn, including artists and characters with less data. The core strategy is data weighting - increasing the weight of data with less data, that is, the number of times it is repeatedly trained in a single epoch, and supplemented with other strategies to help concept learning, such as randomly removing core features of characters. The training parameters for the concept reinforcement stage are exactly the same as those for rough practice.
-
Refinement: This stage uses data with quality ratings of best and amazing to continue fine-tuning the model, freezes the text encoder, enables label randomization algorithm, adds noise offset of 0.0357, and uniformly samples time steps during training. Learning Rate: Constants 2e-6 (unet), 1e-6 (text encoder) Equivalent batch size: 48 Optimizer: AdaFactor (False relative_step, scale_parameter and warmup_init) Noise offset: 0.0357 Time step sampling: uniform distribution (same as normal training) Minimum signal to noise ratio: 5
VI. Terms of Use
-
The model was developed by Nieta Lab : Neta.art Lab - https://civitai.com/user/neta_art
-
Collaborative participants:
-
Chenkin: https://civitai.com/user/Chenkin
-
Bo Dai: https://daibo.info/
-
Terms of Use:
-
The model was developed from ArtiWaifu and AAM XL using Fair AI Public License 1.0-SD
-
If you later modify, merge, or develop the model again, you need to open source the subsequent derived model .
-
VII. Summary and Outlook
Neta Art DiT follow-up training. Stay tuned and test our product for free: https://neta.art .
Bilibili: https://space.bilibili.com/505727005
RED: https://www.xiaohongshu.com/user/profile/63f2ebf2000000001001e206
Twitter: https://twitter.com/netaart_ai
Civitai:https://civitai.com/user/neta_art
描述:
Full report:
https://nieta-art.feishu.cn/wiki/PpwqwVDzjiNE5kkUhRtcEsn6nmh
First release address:
Civitai - https://civitai.com/models/410737
Liblib - https://www.liblib.art/modelinfo/55b06e35dd724862b3524ff00b069fe8
I. Overview
-
We have updated Neta Art XL V2.0. Comparing to version 1.0, we have optimized the character's posture dynamics, further strengthened hand stability, and are very good at telling stories with atmospheric epic scenes.
-
Main motivation:
-
In V1.0, the default poses generated by our characters are relatively fixed, while the new version enhances the sense of dynamics, diverse character camera expressions, and more precise prompt control.
-
On the basis of increasing more stylistic diversity, more stable anatomy has been maintained, especially the probability of having five fingers (instead of four or six) on the hands is now higher!
-
Further training methods for issues such as Rectified Flow and Noise Offset have been better deployed. The current picture has a strong sense of epic, and can now show very bright and dark scenery (as shown in the figure below).
-
{
"prompt": "1girl, full moon, moonlight shadow, cinematic lighting, battle field, fighting armies, castle, warriors around, waving, looking at viewer, Heterochromatic pupil, sitting on a cliff, very dark, epic scene, inferno, cowboy shot, depressed, angel wings, solo, white long hair, wings bangs, beautiful color, amazing quality,",
"negative_prompt": "nsfw, lowres, bad anatomy, bad hands, text, error, missing fingers, extra digit, fewer digits, cropped, worst quality, low quality, normal quality, jpeg artifacts, signature, watermark, username, blurry, artist name, rating: sensitive, low contrast, signature, flexible deformity, abstract, low contrast, ",
"resolution": "1344 x 768",
"guidance_scale": 8,
"num_inference_steps": 28,
"sampler": "Euler a",
"use_lora": null,
"use_upscaler": {
"upscale_method": "bislerp",
"upscaler_strength": 0.65,
"upscale_by": 1.5,
"upscale_cfg": 11,
"new_resolution": "2016 x 1152"
}
}
Tip guide
In Neta V2, we re-trained based on ArtiWaifu Diffusion V1.0 (AWA1). Therefore, we used the prompt order summarized in ArtiWaifu:
Tag order: art style ( by xxx ) - > character ( 1 frieren (sousou no frieren) ) - > race (elf) - > composition (cowboy shot) - > painting style ( impasto ) - > theme (fantasy theme) - > main environment (in the forest, at day) - > background (gradient background) - > action (sitting on ground) - > expression (expressionless) - > main characteristics (white hair) - > other characteristics (Twintails, green eyes, parted lips) - > clothing (wearing a white dress) - > clothing accessories (frills) - > other items (holding a magic wand) - > secondary environment (grass, sunshine) - > aesthetics ( beautiful color , detailed ) - > quality ( best quality) - > secondary description (birds, clouds, butterflies)
[{
"prompt": "(oil-pasto:0.6), half-pasto, anime coloring, 1girl, raiden shogun\(genshin_impact\), genshin_impact, solo, smile, sitting beside window, indoor, classroom, hand rest, japanese school uniform,"
},
{
"prompt": "(line sketch, (black and white, monochrome:1.2), draft, 1girl, solo, march 7th\(honkai: star rail\),honkai: star rail, cowboy shot, medium shot, smile, standing, hand rest,"
},
{
"prompt": "gufeng style, 2.5d, impasto, old withered vines and ancient trees, a murder of crows descending into darkness, a small bridge over gently flowing water, quaint houses nestled by the river, best quality, masterpiece, highres,"
},
{
"prompt": "(2.5d:1.4),(pseudo-impasto:1.2),best quality, masterpiece, ultra-detailed, illustration, detailed light,an extremely delicate and beautiful, incredibly_absurdres, <lora:sdxl_zzz_all_v2:1>,1girl, nicole_demara, solo,crop_top, hair_ribbon, two_side_up, black_ribbon, tube_top, midriff, hairclip, bare_shoulders, black_shorts, cleavage, long_sleeves, cowboy shot, standing, medim shot,"
},
{
"prompt": "pixel art, 1girl, solo, kar98k\(girls' frontline\),girls' frontline, cowboy shot, standing, medim shot,"
}]
Negative prompt: ( worst quality : 1.3), low quality , lowres , messy , abstract, ugly, disfigured, bad anatomy, draft, deformed hands, fused fingers, signature, text, multi views
Sampler parameters: Eular a is recommended as default for 28 + inferences.
Neta Art XL 2.0 still supports a very wide range of CFGs (3-20). But this time we have re-aligned the standard CFG of the model to the normal range of 7-9 for everyone to switch experiments.
II. New style recommendations
We have updated some style activation words and optimized the training. To avoid too much confusion with the original meaning of these words, it is now recommended to add a style suffix when activating.
Classic anime
(Activation word: anime coloring)
Optimize thick impasto
(Activating word: oil-pasto style)
Semi-thick impasto
(Activation word: half-impasto style)
Heavy training 2.5d
(Activation word: 2.5d style)
Neta Art XL 2.0 updates a number of special artists, activated using the by xxx
tag.
by chi4
by hitomio16
by ikky
by ciloranko
by yomu
You can refer to https://civitai.com/models/435207/artiwaifu-diffusion to find more supported artists
III. Better role dynamics
1girl, jack-o' challenge
1girl, upside-down
1boy, harry potter, magic wand, amazing quality
1girl, yoimiya\(genshin impact\), by as109, by antifreeze3, by sho \(sho lwlw\), by junny
Neta Art XL V2.0
Neta Art XL V1.0
Original question
Incoordination of limbs
Not distinguishing hands and feet
Scenes are more templated
V1's stylization is not obvious and more like a fixed character illustration, while V2's characters are very vivid and lively
IV. Epic scenes
1girl, amazing quality, ultra wide shot of Gal Gadot Wonder Woman, holding Mjölnir Hammer, fighting in THOR movie, action-packed, ultra-wide, battle scene, intense, high-quality digital camera, epic cinematic shot, high-resolution digital image, sharp focus, panchromatic film,
super dark, extreme dark, fireflies, unicorn, forest, twilight
Trump, at a public gathering, on stage, flags over head, giving a speech, people screaming, amazing quality
Depth of field in battle scenes
Atmosphere of extremely dark scenes
Dramatic tension
V. Training strategies
Model Training is divided into three stages.
-
Rough practice: Teach models basic knowledge and concepts of the second dimension. Learning Rate: Constants 1e-5 (unet), 7.5e-6 (text encoder) Equivalent batch size: 96 Optimizer: AdaFactor (False relative_step, scale_parameter and warmup_init) Noise-free offset Time step sampling: LogitNormal (ln3,1) Minimum signal to noise ratio: 5 Note: Since the basic model has most of the prior knowledge, this stage is skipped in actual training.
-
Concept Reinforcement: This stage teaches and reinforces all the concepts that the model needs to learn, including artists and characters with less data. The core strategy is data weighting - increasing the weight of data with less data, that is, the number of times it is repeatedly trained in a single epoch, and supplemented with other strategies to help concept learning, such as randomly removing core features of characters. The training parameters for the concept reinforcement stage are exactly the same as those for rough practice.
-
Refinement: This stage uses data with quality ratings of best and amazing to continue fine-tuning the model, freezes the text encoder, enables label randomization algorithm, adds noise offset of 0.0357, and uniformly samples time steps during training. Learning Rate: Constants 2e-6 (unet), 1e-6 (text encoder) Equivalent batch size: 48 Optimizer: AdaFactor (False relative_step, scale_parameter and warmup_init) Noise offset: 0.0357 Time step sampling: uniform distribution (same as normal training) Minimum signal to noise ratio: 5
VI. Terms of Use
-
The model was developed by Pinch Ta Lab : Neta.art Lab - https://civitai.com/user/neta_art
-
Collaborative participants:
-
Chenkin: https://civitai.com/user/Chenkin
-
Bo Dai: https://daibo.info/
-
Terms of Use:
-
The model was developed from ArtiWaifu and AAM XL using Fair AI Public License 1.0-SD
-
If you later modify, merge, or develop the model again, you need to open source the subsequent derived model .
-
VI. Summary and Outlook
Neta Art DiT follow-up training. Stay tuned and test our product for free: http://nieta.art .
Bilibili: https://space.bilibili.com/505727005
RED: https://www.xiaohongshu.com/user/profile/63f2ebf2000000001001e206
Twitter: https://twitter.com/netaart_ai
Civitai:https://civitai.com/user/neta_art
训练词语:
名称: netaArtXL_v20.safetensors
大小 (KB): 6775431
类型: Model
Pickle 扫描结果: Success
Pickle 扫描信息: No Pickle imports
病毒扫描结果: Success